Document Type
Article
Publication Date
2023
Keywords
efficient training, Alzheimer’s disease, deep learning, 3D-to-2D module
Abstract
Three-dimensional convolutional neural networks (3D CNNs) have been widely applied to analyze Alzheimer’s disease (AD) brain images for a better understanding of the disease progress or predicting the conversion from cognitively impaired (CU) or mild cognitive impairment status. It is well-known that training 3D-CNN is computationally expensive and with the potential of overfitting due to the small sample size available in the medical imaging field. Here we proposed a novel 3D-2D approach by converting a 3D brain image to a 2D fused image using a Learnable Weighted Pooling (LWP) method to improve efficient training and maintain comparable model performance. By the 3D-to-2D conversion, the proposed model can easily forward the fused 2D image through a pre-trained 2D model while achieving better performance over different 3D and 2D baselines. In the implementation, we chose to use ResNet34 for feature extraction as it outperformed other 2D CNN backbones. We further showed that the weights of the slices are location-dependent and the model performance relies on the 3D-to-2D fusion view, with the best outcomes from the coronal view. With the new approach, we were able to reduce 75% of the training time and increase the accuracy to 0.88, compared with conventional 3D CNNs, for classifying amyloid-beta PET imaging from the AD patients from the CU participants using the publicly available Alzheimer’s Disease Neuroimaging Initiative dataset. The novel 3D-2D model may have profound implications for timely AD diagnosis in clinical settings in the future.
Digital Object Identifier (DOI)
https://doi.org/10.3390/electronics12020467
Repository Citation
Xing, Xin; Rafique, Muhammad Usman; Liang, Gongbo; Blanton, Hunter; Zhang, Zu; Wang, Chris; Jacobs, Nathan; and Lin, Ai-Ling, "Efficient Training on Alzheimer’s Disease Diagnosis with Learnable Weighted Pooling for 3D PET Brain Image Classification" (2023). Computer Science Faculty Publications. 31.
https://digitalcommons.tamusa.edu/computer_faculty/31
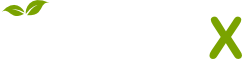
- Citations
- Citation Indexes: 18
- Usage
- Downloads: 95
- Abstract Views: 14
- Captures
- Readers: 29
- Mentions
- News Mentions: 1
Included in
Analytical, Diagnostic and Therapeutic Techniques and Equipment Commons, Electrical and Computer Engineering Commons
Comments
Originally published as:
Xing, X.; Rafique, M.U.; Liang, G.; Blanton, H.; Zhang, Y.; Wang, C.; Jacobs, N.; Lin, A.-L. Efficient Training on Alzheimer’s Disease Diagnosis with Learnable Weighted Pooling for 3D PET Brain Image Classification. Electronics 2023, 12, 467. https://doi.org/10.3390/electronics12020467
This work is licensed under a Creative Commons Attribution 4.0 International License.