Document Type
Article
Publication Date
9-28-2022
Keywords
pneumonia detection; deep learning; lesion localization
Abstract
Pneumonia is a severe inflammation of the lung that could cause serious complications. Chest X-rays (CXRs) are commonly used to make a diagnosis of pneumonia. In this paper, we propose a deep-learning-based method with spatial attention superposition (SAS) and multilayer feature fusion (MFF) to facilitate pneumonia diagnosis based on CXRs. Specifically, an SAS module, which takes advantage of the channel and spatial attention mechanisms, was designed to identify intrinsic imaging features of pneumonia-related lesions and their locations, and an MFF module was designed to harmonize disparate features from different channels and emphasize important information. These two modules were concatenated to extract critical image features serving as the basis for pneumonia diagnosis. We further embedded the proposed modules into a baseline neural network and developed a model called SAS-MFF-YOLO to diagnose pneumonia. To validate the effectiveness of our model, extensive experiments were conducted on two CXR datasets provided by the Radiological Society of North America (RSNA) and the AI Research Institute. SAS-MFF-YOLO achieved a precision of 88.1%, a recall of 98.2% for pneumonia classification and an AP50 of 99% for lesion detection on the AI Research Institute dataset. The visualization of intermediate feature maps showed that our method could facilitate uncovering pneumonia-related lesions in CXRs. Our results demonstrated that our approach could be used to enhance the performance of the overall pneumonia detection on CXR imaging.
Digital Object Identifier (DOI)
https://doi.org/ 10.3390/electronics11193102
Repository Citation
Li, Kang; Zheng, Fengbo; Wu, Panpan; Wang, Qiuyuan; Liang, Gongbo; and Jiang, Lifen, "Improving Pneumonia Classification and Lesion Detection Using Spatial Attention Superposition and Multilayer Feature Fusion" (2022). Computer Science Faculty Publications. 30.
https://digitalcommons.tamusa.edu/computer_faculty/30
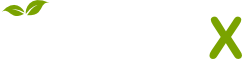
- Citations
- Citation Indexes: 3
- Usage
- Downloads: 92
- Abstract Views: 8
- Captures
- Readers: 9
- Mentions
- News Mentions: 1
Comments
Originally published as:
Li, K., Zheng, F., Wu, P., Wang, Q., Liang, G., & Jiang, L. (2022). Improving Pneumonia Classification and Lesion Detection Using Spatial Attention Superposition and Multilayer Feature Fusion. Electronics, 11(19), 3102.
This is an open access article distributed under the Creative Commons Attribution License which permits unrestricted use, distribution, and reproduction in any medium, provided the original work is properly cited.